Tutorial: optimal binning with binary target under uncertainty¶
The drawback of performing optimal binning given only expected event rates is that variability of event rates in different periods is not taken into account. In this tutorial, we show how scenario-based stochastic programming allows incorporating uncertainty without much difficulty.
[1]:
import matplotlib.pyplot as plt
import numpy as np
import pandas as pd
from scipy import stats
[2]:
from optbinning import OptimalBinning
from optbinning.binning.uncertainty import SBOptimalBinning
Scenario generation¶
We generate three scenarios, all equally likely, aiming to represent three economic scenarios severity using the customer’s score variable, for instance.
Scenario 0 - Normal (Realistic): A low customer’ score has a higher event rate (default rate, churn, etc) than a high customer’s score. The population corresponding to non-event and event are reasonably separated.
[3]:
N0 = int(1e5)
xe = stats.beta(a=4, b=15).rvs(size=N0, random_state=42)
ye = stats.bernoulli(p=0.7).rvs(size=N0, random_state=42)
xn = stats.beta(a=6, b=8).rvs(size=N0, random_state=42)
yn = stats.bernoulli(p=0.2).rvs(size=N0, random_state=42)
x0 = np.concatenate((xn, xe), axis=0)
y0 = np.concatenate((yn, ye), axis=0)
[4]:
def plot_distribution(x, y):
plt.hist(x[y == 0], label="n_nonevent", color="b", alpha=0.5)
plt.hist(x[y == 1], label="n_event", color="r", alpha=0.5)
plt.legend()
plt.show()
[5]:
plot_distribution(x0, y0)
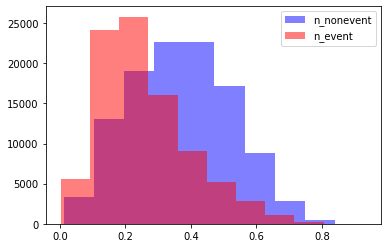
Scenario 1: Good (Optimistic): A low customer’ score has a much higher event rate (default rate, churn, etc) than a high customer’s score. The population corresponding to non-event and event rate are very well separated, showing minimum overlap regions.
[6]:
N1 = int(5e4)
xe = stats.beta(a=25, b=50).rvs(size=N1, random_state=42)
ye = stats.bernoulli(p=0.9).rvs(size=N1, random_state=42)
xn = stats.beta(a=22, b=25).rvs(size=N1, random_state=42)
yn = stats.bernoulli(p=0.05).rvs(size=N1, random_state=42)
x1 = np.concatenate((xn, xe), axis=0)
y1 = np.concatenate((yn, ye), axis=0)
[7]:
plot_distribution(x1, y1)
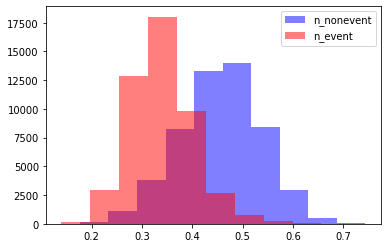
Scenario 2: Bad (Pessimistic): Customer’s behavior cannot be accurately segmented, and a general increase in event rates is exhibited. The populations corresponding to non-event and event are practically overlapped.
[8]:
N2 = int(5e4)
xe = stats.beta(a=4, b=6).rvs(size=N2, random_state=42)
ye = stats.bernoulli(p=0.7).rvs(size=N2, random_state=42)
xn = stats.beta(a=8, b=10).rvs(size=N2, random_state=42)
yn = stats.bernoulli(p=0.4).rvs(size=N2, random_state=42)
x2 = np.concatenate((xn, xe), axis=0)
y2 = np.concatenate((yn, ye), axis=0)
[9]:
plot_distribution(x2, y2)
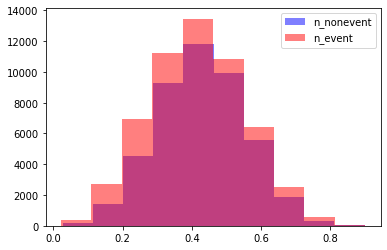
Scenario-based stochastic optimal binning¶
Prepare scenarios data and instantiate an SBOptimalBinning
object class. We set a descending monotonicity constraint with respect to event rate and a minimum bin size.
[10]:
X = [x0, x1, x2]
Y = [y0, y1, y2]
[11]:
sboptb = SBOptimalBinning(monotonic_trend="descending", min_bin_size=0.05)
sboptb.fit(X, Y)
[11]:
SBOptimalBinning(min_bin_size=0.05, monotonic_trend='descending')
[12]:
sboptb.status
[12]:
'OPTIMAL'
We obtain “only” three splits guaranteeing feasibility for each scenario.
[13]:
sboptb.splits
[13]:
array([0.28578988, 0.36384453, 0.43260857])
[14]:
sboptb.information(print_level=2)
optbinning (Version 0.19.0)
Copyright (c) 2019-2024 Guillermo Navas-Palencia, Apache License 2.0
Begin options
name * d
prebinning_method cart * d
max_n_prebins 20 * d
min_prebin_size 0.05 * d
min_n_bins no * d
max_n_bins no * d
min_bin_size 0.05 * U
max_bin_size no * d
monotonic_trend descending * U
min_event_rate_diff 0 * d
max_pvalue no * d
max_pvalue_policy consecutive * d
class_weight no * d
user_splits no * d
user_splits_fixed no * d
special_codes no * d
split_digits no * d
time_limit 100 * d
verbose False * d
End options
Name : UNKNOWN
Status : OPTIMAL
Pre-binning statistics
Number of pre-bins 16
Number of refinements 1
Solver statistics
Type cp
Number of booleans 40
Number of branches 91
Number of conflicts 1
Objective value 2736534
Best objective bound 2736534
Timing
Total time 1.21 sec
Pre-processing 0.01 sec ( 0.90%)
Pre-binning 0.70 sec ( 58.18%)
Solver 0.49 sec ( 40.72%)
model generation 0.44 sec ( 90.22%)
optimizer 0.05 sec ( 9.78%)
Post-processing 0.00 sec ( 0.10%)
The binning table¶
As other optimal binning algorithms in OptBinning, SBOptimalBinning
also returns a binning table displaying the binned data considering all scenarios.
[15]:
sboptb.binning_table.build()
[15]:
Bin | Count | Count (%) | Non-event | Event | Event rate | WoE | IV | JS | |
---|---|---|---|---|---|---|---|---|---|
0 | (-inf, 0.29) | 119678 | 0.299195 | 42005 | 77673 | 0.649017 | -0.688603 | 0.138209 | 0.016943 |
1 | [0.29, 0.36) | 79729 | 0.199323 | 32837 | 46892 | 0.588142 | -0.430175 | 0.036613 | 0.004542 |
2 | [0.36, 0.43) | 68378 | 0.170945 | 39045 | 29333 | 0.428983 | 0.212118 | 0.007633 | 0.000952 |
3 | [0.43, inf) | 132215 | 0.330537 | 93498 | 38717 | 0.292834 | 0.807778 | 0.201811 | 0.024562 |
4 | Special | 0 | 0.000000 | 0 | 0 | 0.000000 | 0.000000 | 0.000000 | 0.000000 |
5 | Missing | 0 | 0.000000 | 0 | 0 | 0.000000 | 0.000000 | 0.000000 | 0.000000 |
Totals | 400000 | 1.000000 | 207385 | 192615 | 0.481538 | 0.384266 | 0.046999 |
[16]:
sboptb.binning_table.plot(metric="event_rate")
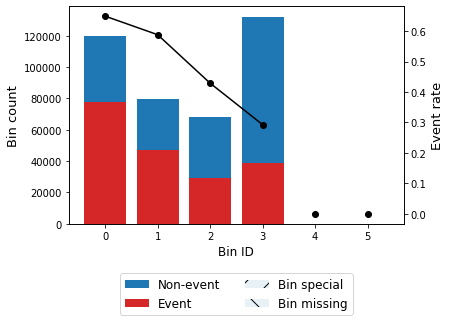
[17]:
sboptb.binning_table.analysis()
---------------------------------------------
OptimalBinning: Binary Binning Table Analysis
---------------------------------------------
General metrics
Gini index 0.33117510
IV (Jeffrey) 0.38426602
JS (Jensen-Shannon) 0.04699884
Hellinger 0.04750871
Triangular 0.18408357
KS 0.28582022
HHI 0.26772434
HHI (normalized) 0.12126921
Cramer's V 0.30285954
Quality score 0.87798527
Monotonic trend descending
Significance tests
Bin A Bin B t-statistic p-value P[A > B] P[B > A]
0 1 756.303469 1.709260e-166 1.0 1.110223e-16
1 2 3732.973381 0.000000e+00 1.0 1.110223e-16
2 3 3726.998391 0.000000e+00 1.0 1.110223e-16
Expected value solution (EVS)¶
The expected value solution is calculated with the normal (expected) scenario.
[18]:
optb = OptimalBinning(monotonic_trend="descending", min_bin_size=0.05)
optb.fit(x0, y0)
[18]:
OptimalBinning(min_bin_size=0.05, monotonic_trend='descending')
[19]:
optb.binning_table.build()
[19]:
Bin | Count | Count (%) | Non-event | Event | Event rate | WoE | IV | JS | |
---|---|---|---|---|---|---|---|---|---|
0 | (-inf, 0.10) | 10255 | 0.051275 | 3061 | 7194 | 0.701511 | -1.054853 | 0.054945 | 0.006566 |
1 | [0.10, 0.14) | 12519 | 0.062595 | 3911 | 8608 | 0.687595 | -0.989246 | 0.059422 | 0.007139 |
2 | [0.14, 0.18) | 18333 | 0.091665 | 6065 | 12268 | 0.669176 | -0.904807 | 0.073418 | 0.008877 |
3 | [0.18, 0.20) | 13631 | 0.068155 | 4884 | 8747 | 0.641699 | -0.783094 | 0.041320 | 0.005037 |
4 | [0.20, 0.23) | 14606 | 0.073030 | 5684 | 8922 | 0.610845 | -0.651212 | 0.030891 | 0.003795 |
5 | [0.23, 0.27) | 17995 | 0.089975 | 8043 | 9952 | 0.553043 | -0.413319 | 0.015470 | 0.001920 |
6 | [0.27, 0.30) | 13047 | 0.065235 | 6672 | 6375 | 0.488618 | -0.154812 | 0.001572 | 0.000196 |
7 | [0.30, 0.35) | 18825 | 0.094125 | 11158 | 7667 | 0.407278 | 0.174884 | 0.002847 | 0.000355 |
8 | [0.35, 0.39) | 16401 | 0.082005 | 10903 | 5498 | 0.335223 | 0.484306 | 0.018430 | 0.002282 |
9 | [0.39, 0.44) | 18759 | 0.093795 | 13688 | 5071 | 0.270324 | 0.792634 | 0.053994 | 0.006578 |
10 | [0.44, 0.49) | 14549 | 0.072745 | 11273 | 3276 | 0.225170 | 1.035440 | 0.068446 | 0.008193 |
11 | [0.49, 0.54) | 11019 | 0.055095 | 8697 | 2322 | 0.210727 | 1.120202 | 0.059684 | 0.007093 |
12 | [0.54, 0.60) | 10030 | 0.050150 | 7957 | 2073 | 0.206680 | 1.144708 | 0.056454 | 0.006695 |
13 | [0.60, inf) | 10031 | 0.050155 | 7988 | 2043 | 0.203669 | 1.163174 | 0.058080 | 0.006877 |
14 | Special | 0 | 0.000000 | 0 | 0 | 0.000000 | 0.000000 | 0.000000 | 0.000000 |
15 | Missing | 0 | 0.000000 | 0 | 0 | 0.000000 | 0.000000 | 0.000000 | 0.000000 |
Totals | 200000 | 1.000000 | 109984 | 90016 | 0.450080 | 0.594974 | 0.071603 |
[20]:
optb.binning_table.plot(metric="event_rate")
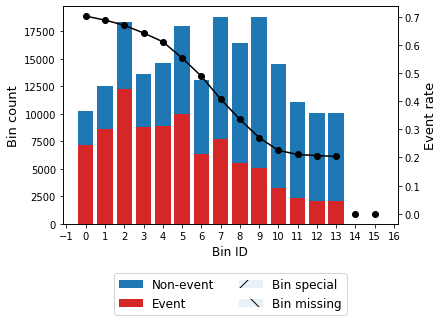
[21]:
optb.binning_table.analysis()
---------------------------------------------
OptimalBinning: Binary Binning Table Analysis
---------------------------------------------
General metrics
Gini index 0.42141055
IV (Jeffrey) 0.59497411
JS (Jensen-Shannon) 0.07160267
Hellinger 0.07295186
Triangular 0.27638899
KS 0.34108533
HHI 0.07501900
HHI (normalized) 0.01335360
Cramer's V 0.36927482
Quality score 0.16335319
Monotonic trend descending
Significance tests
Bin A Bin B t-statistic p-value P[A > B] P[B > A]
0 1 5.139745 2.338408e-02 0.988706 1.129409e-02
1 2 11.534993 6.829832e-04 0.999721 2.787731e-04
2 3 26.208899 3.064073e-07 1.000000 7.445353e-09
3 4 28.661681 8.619251e-08 1.000000 4.436704e-09
4 5 110.500800 7.611468e-26 1.000000 1.110223e-16
5 6 125.906119 3.223792e-29 1.000000 1.110223e-16
6 7 206.865709 6.632897e-47 1.000000 1.110223e-16
7 8 194.419542 3.449032e-44 1.000000 1.110223e-16
8 9 175.309976 5.122903e-40 1.000000 1.110223e-16
9 10 88.957203 4.034468e-21 1.000000 1.110223e-16
10 11 7.648694 5.681344e-03 0.997558 2.442113e-03
11 12 0.520543 4.706103e-01 0.764881 2.351195e-01
12 13 0.278879 5.974371e-01 0.701329 2.986709e-01
Scenario analysis¶
Scenario 0 - Normal (Realistic)¶
[22]:
bt0 = sboptb.binning_table_scenario(scenario_id=0)
bt0.build()
[22]:
Bin | Count | Count (%) | Non-event | Event | Event rate | WoE | IV | JS | |
---|---|---|---|---|---|---|---|---|---|
0 | (-inf, 0.29) | 93851 | 0.469255 | 34903 | 58948 | 0.628102 | -0.724430 | 0.244506 | 0.029912 |
1 | [0.29, 0.36) | 32141 | 0.160705 | 18945 | 13196 | 0.410566 | 0.161279 | 0.004138 | 0.000517 |
2 | [0.36, 0.43) | 24488 | 0.122440 | 17289 | 7199 | 0.293981 | 0.675781 | 0.052184 | 0.006402 |
3 | [0.43, inf) | 49520 | 0.247600 | 38847 | 10673 | 0.215529 | 1.091566 | 0.256123 | 0.030515 |
4 | Special | 0 | 0.000000 | 0 | 0 | 0.000000 | 0.000000 | 0.000000 | 0.000000 |
5 | Missing | 0 | 0.000000 | 0 | 0 | 0.000000 | 0.000000 | 0.000000 | 0.000000 |
Totals | 200000 | 1.000000 | 109984 | 90016 | 0.450080 | 0.556952 | 0.067345 |
[23]:
bt0.plot(metric="event_rate")
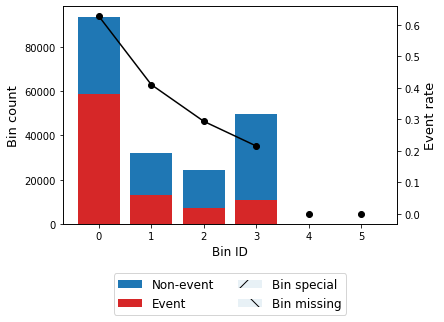
[24]:
optb0 = OptimalBinning(monotonic_trend="descending", min_bin_size=0.05)
optb0.fit(x0, y0)
[24]:
OptimalBinning(min_bin_size=0.05, monotonic_trend='descending')
[25]:
optb0.binning_table.build()
[25]:
Bin | Count | Count (%) | Non-event | Event | Event rate | WoE | IV | JS | |
---|---|---|---|---|---|---|---|---|---|
0 | (-inf, 0.10) | 10255 | 0.051275 | 3061 | 7194 | 0.701511 | -1.054853 | 0.054945 | 0.006566 |
1 | [0.10, 0.14) | 12519 | 0.062595 | 3911 | 8608 | 0.687595 | -0.989246 | 0.059422 | 0.007139 |
2 | [0.14, 0.18) | 18333 | 0.091665 | 6065 | 12268 | 0.669176 | -0.904807 | 0.073418 | 0.008877 |
3 | [0.18, 0.20) | 13631 | 0.068155 | 4884 | 8747 | 0.641699 | -0.783094 | 0.041320 | 0.005037 |
4 | [0.20, 0.23) | 14606 | 0.073030 | 5684 | 8922 | 0.610845 | -0.651212 | 0.030891 | 0.003795 |
5 | [0.23, 0.27) | 17995 | 0.089975 | 8043 | 9952 | 0.553043 | -0.413319 | 0.015470 | 0.001920 |
6 | [0.27, 0.30) | 13047 | 0.065235 | 6672 | 6375 | 0.488618 | -0.154812 | 0.001572 | 0.000196 |
7 | [0.30, 0.35) | 18825 | 0.094125 | 11158 | 7667 | 0.407278 | 0.174884 | 0.002847 | 0.000355 |
8 | [0.35, 0.39) | 16401 | 0.082005 | 10903 | 5498 | 0.335223 | 0.484306 | 0.018430 | 0.002282 |
9 | [0.39, 0.44) | 18759 | 0.093795 | 13688 | 5071 | 0.270324 | 0.792634 | 0.053994 | 0.006578 |
10 | [0.44, 0.49) | 14549 | 0.072745 | 11273 | 3276 | 0.225170 | 1.035440 | 0.068446 | 0.008193 |
11 | [0.49, 0.54) | 11019 | 0.055095 | 8697 | 2322 | 0.210727 | 1.120202 | 0.059684 | 0.007093 |
12 | [0.54, 0.60) | 10030 | 0.050150 | 7957 | 2073 | 0.206680 | 1.144708 | 0.056454 | 0.006695 |
13 | [0.60, inf) | 10031 | 0.050155 | 7988 | 2043 | 0.203669 | 1.163174 | 0.058080 | 0.006877 |
14 | Special | 0 | 0.000000 | 0 | 0 | 0.000000 | 0.000000 | 0.000000 | 0.000000 |
15 | Missing | 0 | 0.000000 | 0 | 0 | 0.000000 | 0.000000 | 0.000000 | 0.000000 |
Totals | 200000 | 1.000000 | 109984 | 90016 | 0.450080 | 0.594974 | 0.071603 |
[26]:
optb0.binning_table.plot(metric="event_rate")
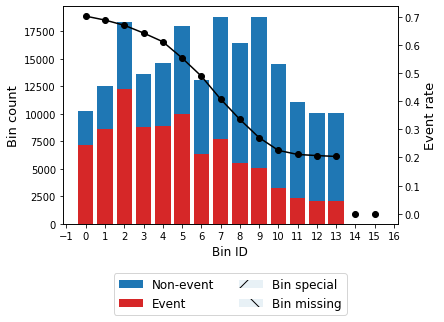
Apply expected value solution to scenario 0.
[27]:
evs_optb0 = OptimalBinning(user_splits=optb.splits)
evs_optb0.fit(x0, y0)
[27]:
OptimalBinning(user_splits=array([0.1008646 , 0.13640077, 0.17635711, 0.20390539, 0.23334569,
0.27135116, 0.30051835, 0.34623086, 0.38964605, 0.44464479,
0.49356021, 0.53990114, 0.59801421]))
[28]:
evs_optb0.binning_table.build()
[28]:
Bin | Count | Count (%) | Non-event | Event | Event rate | WoE | IV | JS | |
---|---|---|---|---|---|---|---|---|---|
0 | (-inf, 0.10) | 10255 | 0.051275 | 3061 | 7194 | 0.701511 | -1.054853 | 0.054945 | 0.006566 |
1 | [0.10, 0.14) | 12519 | 0.062595 | 3911 | 8608 | 0.687595 | -0.989246 | 0.059422 | 0.007139 |
2 | [0.14, 0.18) | 18333 | 0.091665 | 6065 | 12268 | 0.669176 | -0.904807 | 0.073418 | 0.008877 |
3 | [0.18, 0.20) | 13631 | 0.068155 | 4884 | 8747 | 0.641699 | -0.783094 | 0.041320 | 0.005037 |
4 | [0.20, 0.23) | 14606 | 0.073030 | 5684 | 8922 | 0.610845 | -0.651212 | 0.030891 | 0.003795 |
5 | [0.23, 0.27) | 17995 | 0.089975 | 8043 | 9952 | 0.553043 | -0.413319 | 0.015470 | 0.001920 |
6 | [0.27, 0.30) | 13047 | 0.065235 | 6672 | 6375 | 0.488618 | -0.154812 | 0.001572 | 0.000196 |
7 | [0.30, 0.35) | 18825 | 0.094125 | 11158 | 7667 | 0.407278 | 0.174884 | 0.002847 | 0.000355 |
8 | [0.35, 0.39) | 16401 | 0.082005 | 10903 | 5498 | 0.335223 | 0.484306 | 0.018430 | 0.002282 |
9 | [0.39, 0.44) | 18759 | 0.093795 | 13688 | 5071 | 0.270324 | 0.792634 | 0.053994 | 0.006578 |
10 | [0.44, 0.49) | 14549 | 0.072745 | 11273 | 3276 | 0.225170 | 1.035440 | 0.068446 | 0.008193 |
11 | [0.49, 0.54) | 11019 | 0.055095 | 8697 | 2322 | 0.210727 | 1.120202 | 0.059684 | 0.007093 |
12 | [0.54, 0.60) | 10030 | 0.050150 | 7957 | 2073 | 0.206680 | 1.144708 | 0.056454 | 0.006695 |
13 | [0.60, inf) | 10031 | 0.050155 | 7988 | 2043 | 0.203669 | 1.163174 | 0.058080 | 0.006877 |
14 | Special | 0 | 0.000000 | 0 | 0 | 0.000000 | 0.000000 | 0.000000 | 0.000000 |
15 | Missing | 0 | 0.000000 | 0 | 0 | 0.000000 | 0.000000 | 0.000000 | 0.000000 |
Totals | 200000 | 1.000000 | 109984 | 90016 | 0.450080 | 0.594974 | 0.071603 |
[29]:
evs_optb0.binning_table.plot(metric="event_rate")
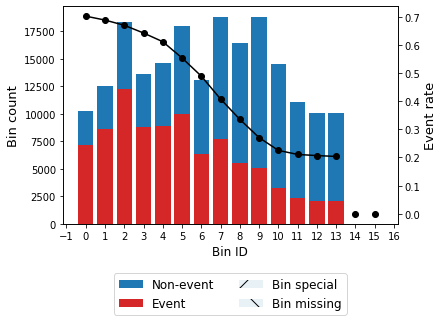
The expected value solution applied to scenarion 0 does not satisfy the min_bin_size
constraint, hence the solution is not feasible.
[30]:
EVS_0 = 0.594974
Scenario 1: Good (Optimistic)
[31]:
bt1 = sboptb.binning_table_scenario(scenario_id=1)
bt1.build()
[31]:
Bin | Count | Count (%) | Non-event | Event | Event rate | WoE | IV | JS | |
---|---|---|---|---|---|---|---|---|---|
0 | (-inf, 0.29) | 9840 | 0.09840 | 1126 | 8714 | 0.885569 | -2.146624 | 0.347828 | 0.036679 |
1 | [0.29, 0.36) | 29807 | 0.29807 | 5902 | 23905 | 0.801993 | -1.499161 | 0.586072 | 0.067087 |
2 | [0.36, 0.43) | 24262 | 0.24262 | 12658 | 11604 | 0.478279 | -0.013425 | 0.000044 | 0.000005 |
3 | [0.43, inf) | 36091 | 0.36091 | 32821 | 3270 | 0.090604 | 2.205914 | 1.226988 | 0.128301 |
4 | Special | 0 | 0.00000 | 0 | 0 | 0.000000 | 0.000000 | 0.000000 | 0.000000 |
5 | Missing | 0 | 0.00000 | 0 | 0 | 0.000000 | 0.000000 | 0.000000 | 0.000000 |
Totals | 100000 | 1.00000 | 52507 | 47493 | 0.474930 | 2.160931 | 0.232072 |
[32]:
bt1.plot(metric="event_rate")
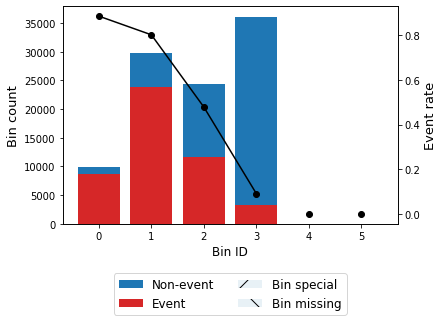
[33]:
optb1 = OptimalBinning(monotonic_trend="descending", min_bin_size=0.05)
optb1.fit(x1, y1)
[33]:
OptimalBinning(min_bin_size=0.05, monotonic_trend='descending')
[34]:
optb1.binning_table.build()
[34]:
Bin | Count | Count (%) | Non-event | Event | Event rate | WoE | IV | JS | |
---|---|---|---|---|---|---|---|---|---|
0 | (-inf, 0.28) | 8209 | 0.08209 | 908 | 7301 | 0.889390 | -2.184886 | 0.298095 | 0.031264 |
1 | [0.28, 0.30) | 5545 | 0.05545 | 738 | 4807 | 0.866907 | -1.974249 | 0.172075 | 0.018581 |
2 | [0.30, 0.31) | 5186 | 0.05186 | 777 | 4409 | 0.850174 | -1.836327 | 0.143301 | 0.015756 |
3 | [0.31, 0.33) | 5837 | 0.05837 | 956 | 4881 | 0.836217 | -1.730712 | 0.146359 | 0.016307 |
4 | [0.33, 0.34) | 5176 | 0.05176 | 1077 | 4099 | 0.791924 | -1.436928 | 0.094544 | 0.010896 |
5 | [0.34, 0.36) | 7055 | 0.07055 | 1760 | 5295 | 0.750532 | -1.201813 | 0.093706 | 0.011056 |
6 | [0.36, 0.38) | 8537 | 0.08537 | 2882 | 5655 | 0.662411 | -0.774420 | 0.049704 | 0.006062 |
7 | [0.38, 0.40) | 6189 | 0.06189 | 2802 | 3387 | 0.547261 | -0.289975 | 0.005205 | 0.000648 |
8 | [0.40, 0.41) | 5058 | 0.05058 | 2862 | 2196 | 0.434164 | 0.164519 | 0.001360 | 0.000170 |
9 | [0.41, 0.44) | 8246 | 0.08246 | 5781 | 2465 | 0.298933 | 0.752021 | 0.043766 | 0.005345 |
10 | [0.44, 0.45) | 5253 | 0.05253 | 4321 | 932 | 0.177422 | 1.433545 | 0.089840 | 0.010358 |
11 | [0.45, 0.47) | 5009 | 0.05009 | 4420 | 589 | 0.117588 | 1.915105 | 0.137461 | 0.014960 |
12 | [0.47, 0.49) | 5204 | 0.05204 | 4780 | 424 | 0.081476 | 2.322098 | 0.190662 | 0.019603 |
13 | [0.49, 0.53) | 8825 | 0.08825 | 8283 | 542 | 0.061416 | 2.626330 | 0.384332 | 0.037733 |
14 | [0.53, 0.56) | 5061 | 0.05061 | 4807 | 254 | 0.050188 | 2.840130 | 0.244824 | 0.023237 |
15 | [0.56, inf) | 5610 | 0.05610 | 5353 | 257 | 0.045811 | 2.935972 | 0.283430 | 0.026488 |
16 | Special | 0 | 0.00000 | 0 | 0 | 0.000000 | 0.000000 | 0.000000 | 0.000000 |
17 | Missing | 0 | 0.00000 | 0 | 0 | 0.000000 | 0.000000 | 0.000000 | 0.000000 |
Totals | 100000 | 1.00000 | 52507 | 47493 | 0.474930 | 2.378665 | 0.248465 |
[35]:
optb1.binning_table.plot(metric="event_rate")
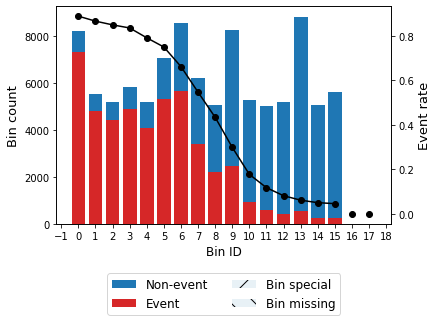
Apply expected value solution to scenario 1.
[36]:
evs_optb1 = OptimalBinning(user_splits=optb.splits)
evs_optb1.fit(x1, y1)
[36]:
OptimalBinning(user_splits=array([0.1008646 , 0.13640077, 0.17635711, 0.20390539, 0.23334569,
0.27135116, 0.30051835, 0.34623086, 0.38964605, 0.44464479,
0.49356021, 0.53990114, 0.59801421]))
[37]:
evs_optb1.binning_table.build()
[37]:
Bin | Count | Count (%) | Non-event | Event | Event rate | WoE | IV | JS | |
---|---|---|---|---|---|---|---|---|---|
0 | (-inf, 0.20) | 247 | 0.00247 | 26 | 221 | 0.894737 | -2.240430 | 0.009316 | 0.000969 |
1 | [0.20, 0.23) | 1092 | 0.01092 | 118 | 974 | 0.891941 | -2.211091 | 0.040377 | 0.004219 |
2 | [0.23, 0.27) | 5037 | 0.05037 | 566 | 4471 | 0.887632 | -2.167137 | 0.180654 | 0.018995 |
3 | [0.27, 0.30) | 7918 | 0.07918 | 1019 | 6899 | 0.871306 | -2.012919 | 0.253339 | 0.027214 |
4 | [0.30, 0.35) | 18126 | 0.18126 | 3313 | 14813 | 0.817224 | -1.598015 | 0.397590 | 0.045005 |
5 | [0.35, 0.39) | 17091 | 0.17091 | 5742 | 11349 | 0.664034 | -0.781686 | 0.101310 | 0.012351 |
6 | [0.39, 0.44) | 18095 | 0.18095 | 11857 | 6238 | 0.344736 | 0.541895 | 0.051194 | 0.006322 |
7 | [0.44, 0.49) | 14295 | 0.14295 | 12739 | 1556 | 0.108849 | 2.002186 | 0.420164 | 0.045199 |
8 | [0.49, 0.54) | 10111 | 0.10111 | 9523 | 588 | 0.058154 | 2.684374 | 0.453620 | 0.044133 |
9 | [0.54, 0.60) | 6215 | 0.06215 | 5918 | 297 | 0.047788 | 2.891658 | 0.307832 | 0.028976 |
10 | [0.60, inf) | 1773 | 0.01773 | 1686 | 87 | 0.049069 | 2.863842 | 0.086712 | 0.008199 |
11 | Special | 0 | 0.00000 | 0 | 0 | 0.000000 | 0.000000 | 0.000000 | 0.000000 |
12 | Missing | 0 | 0.00000 | 0 | 0 | 0.000000 | 0.000000 | 0.000000 | 0.000000 |
Totals | 100000 | 1.00000 | 52507 | 47493 | 0.474930 | 2.302108 | 0.241582 |
[38]:
evs_optb1.binning_table.plot(metric="event_rate")
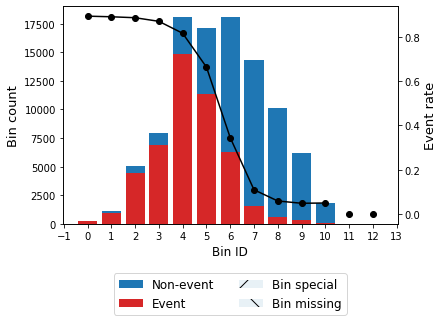
[39]:
evs_optb1.binning_table.analysis()
---------------------------------------------
OptimalBinning: Binary Binning Table Analysis
---------------------------------------------
General metrics
Gini index 0.72566718
IV (Jeffrey) 2.30210757
JS (Jensen-Shannon) 0.24158211
Hellinger 0.26182242
Triangular 0.84830395
KS 0.61004329
HHI 0.13857518
HHI (normalized) 0.06678978
Cramer's V 0.64902999
Quality score 0.00000000
Monotonic trend valley
Significance tests
Bin A Bin B t-statistic p-value P[A > B] P[B > A]
0 1 0.016401 8.980961e-01 0.566231 4.337689e-01
1 2 0.168135 6.817748e-01 0.666387 3.336129e-01
2 3 7.641448 5.704212e-03 0.997457 2.543322e-03
3 4 116.236493 4.218674e-27 1.000000 1.110223e-16
4 5 1080.747496 5.050568e-237 1.000000 1.110223e-16
5 6 3584.325798 0.000000e+00 1.000000 1.110223e-16
6 7 2431.847481 0.000000e+00 1.000000 1.110223e-16
7 8 189.938108 3.279750e-43 1.000000 1.110223e-16
8 9 8.068486 4.504174e-03 0.998346 1.654368e-03
9 10 0.049526 8.238907e-01 0.420232 5.797684e-01
The expected value solution applied to scenario 1 satisfies neither the min_bin_size
constraint nor the monotonicity constraint, hence the solution is not feasible.
[40]:
EVS_1 = -np.inf
Scenario 2: Bad (Pessimistic)
[41]:
bt2 = sboptb.binning_table_scenario(scenario_id=2)
bt2.build()
[41]:
Bin | Count | Count (%) | Non-event | Event | Event rate | WoE | IV | JS | |
---|---|---|---|---|---|---|---|---|---|
0 | (-inf, 0.29) | 15987 | 0.15987 | 5976 | 10011 | 0.626196 | -0.310979 | 1.509941e-02 | 1.879858e-03 |
1 | [0.29, 0.36) | 17781 | 0.17781 | 7990 | 9791 | 0.550644 | 0.001682 | 5.028570e-07 | 6.285711e-08 |
2 | [0.36, 0.43) | 19628 | 0.19628 | 9098 | 10530 | 0.536479 | 0.058781 | 6.800268e-04 | 8.499112e-05 |
3 | [0.43, inf) | 46604 | 0.46604 | 21830 | 24774 | 0.531585 | 0.078445 | 2.877876e-03 | 3.596423e-04 |
4 | Special | 0 | 0.00000 | 0 | 0 | 0.000000 | 0.000000 | 0.000000e+00 | 0.000000e+00 |
5 | Missing | 0 | 0.00000 | 0 | 0 | 0.000000 | 0.000000 | 0.000000e+00 | 0.000000e+00 |
Totals | 100000 | 1.00000 | 44894 | 55106 | 0.551060 | 1.865782e-02 | 2.324554e-03 |
[42]:
bt2.plot(metric="event_rate")
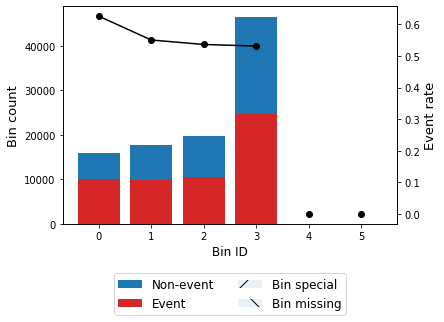
[43]:
optb2 = OptimalBinning(monotonic_trend="descending", min_bin_size=0.05)
optb2.fit(x2, y2)
[43]:
OptimalBinning(min_bin_size=0.05, monotonic_trend='descending')
[44]:
optb2.binning_table.build()
[44]:
Bin | Count | Count (%) | Non-event | Event | Event rate | WoE | IV | JS | |
---|---|---|---|---|---|---|---|---|---|
0 | (-inf, 0.23) | 7556 | 0.07556 | 2543 | 5013 | 0.663446 | -0.473736 | 0.016261 | 0.002014 |
1 | [0.23, 0.29) | 9657 | 0.09657 | 3918 | 5739 | 0.594284 | -0.176749 | 0.002982 | 0.000372 |
2 | [0.29, 0.33) | 8559 | 0.08559 | 3801 | 4758 | 0.555906 | -0.019609 | 0.000033 | 0.000004 |
3 | [0.33, 0.39) | 15848 | 0.15848 | 7234 | 8614 | 0.543539 | 0.030358 | 0.000146 | 0.000018 |
4 | [0.39, inf) | 58380 | 0.58380 | 27398 | 30982 | 0.530695 | 0.082018 | 0.003941 | 0.000493 |
5 | Special | 0 | 0.00000 | 0 | 0 | 0.000000 | 0.000000 | 0.000000 | 0.000000 |
6 | Missing | 0 | 0.00000 | 0 | 0 | 0.000000 | 0.000000 | 0.000000 | 0.000000 |
Totals | 100000 | 1.00000 | 44894 | 55106 | 0.551060 | 0.023364 | 0.002901 |
[45]:
optb2.binning_table.plot(metric="event_rate")
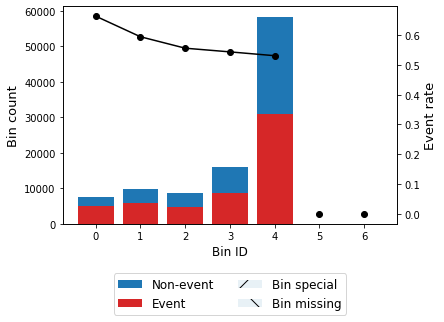
Apply expected value solution to scenario 2.
[46]:
evs_optb2 = OptimalBinning(user_splits=optb.splits)
evs_optb2.fit(x2, y2)
[46]:
OptimalBinning(user_splits=array([0.1008646 , 0.13640077, 0.17635711, 0.20390539, 0.23334569,
0.27135116, 0.30051835, 0.34623086, 0.38964605, 0.44464479,
0.49356021, 0.53990114, 0.59801421]))
[47]:
evs_optb2.binning_table.build()
[47]:
Bin | Count | Count (%) | Non-event | Event | Event rate | WoE | IV | JS | |
---|---|---|---|---|---|---|---|---|---|
0 | (-inf, 0.14) | 1292 | 0.01292 | 405 | 887 | 0.686533 | -0.579003 | 0.004096 | 5.050214e-04 |
1 | [0.14, 0.18) | 1850 | 0.01850 | 598 | 1252 | 0.676757 | -0.533952 | 0.005019 | 6.200181e-04 |
2 | [0.18, 0.20) | 2002 | 0.02002 | 709 | 1293 | 0.645854 | -0.395910 | 0.003037 | 3.771741e-04 |
3 | [0.20, 0.23) | 2944 | 0.02944 | 1049 | 1895 | 0.643682 | -0.386427 | 0.004259 | 5.291176e-04 |
4 | [0.23, 0.27) | 5326 | 0.05326 | 2134 | 3192 | 0.599324 | -0.197695 | 0.002054 | 2.563524e-04 |
5 | [0.27, 0.30) | 5390 | 0.05390 | 2291 | 3099 | 0.574954 | -0.097137 | 0.000506 | 6.318381e-05 |
6 | [0.30, 0.35) | 10414 | 0.10414 | 4667 | 5747 | 0.551853 | -0.003207 | 0.000001 | 1.338664e-07 |
7 | [0.35, 0.39) | 11782 | 0.11782 | 5375 | 6407 | 0.543796 | 0.029322 | 0.000101 | 1.267992e-05 |
8 | [0.39, 0.44) | 15901 | 0.15901 | 7509 | 8392 | 0.527766 | 0.093778 | 0.001404 | 1.754450e-04 |
9 | [0.44, 0.54) | 23757 | 0.23757 | 11416 | 12341 | 0.519468 | 0.127043 | 0.003854 | 4.814509e-04 |
10 | [0.54, 0.60) | 9639 | 0.09639 | 4529 | 5110 | 0.530138 | 0.084256 | 0.000687 | 8.582857e-05 |
11 | [0.60, inf) | 9703 | 0.09703 | 4212 | 5491 | 0.565907 | -0.060218 | 0.000351 | 4.382723e-05 |
12 | Special | 0 | 0.00000 | 0 | 0 | 0.000000 | 0.000000 | 0.000000 | 0.000000e+00 |
13 | Missing | 0 | 0.00000 | 0 | 0 | 0.000000 | 0.000000 | 0.000000 | 0.000000e+00 |
Totals | 100000 | 1.00000 | 44894 | 55106 | 0.551060 | 0.025370 | 3.150233e-03 |
[48]:
evs_optb2.binning_table.plot(metric="event_rate")
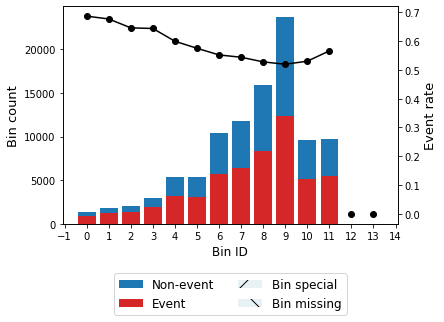
[49]:
evs_optb2.binning_table.analysis()
---------------------------------------------
OptimalBinning: Binary Binning Table Analysis
---------------------------------------------
General metrics
Gini index 0.07657686
IV (Jeffrey) 0.02536981
JS (Jensen-Shannon) 0.00315023
Hellinger 0.00316066
Triangular 0.01251904
KS 0.05109803
HHI 0.13267476
HHI (normalized) 0.06595743
Cramer's V 0.07812501
Quality score 0.00318975
Monotonic trend valley
Significance tests
Bin A Bin B t-statistic p-value P[A > B] P[B > A]
0 1 0.334525 5.630065e-01 7.193040e-01 0.280696
1 2 4.095897 4.298741e-02 9.789856e-01 0.021014
2 3 0.024540 8.755193e-01 5.627139e-01 0.437286
3 4 15.757594 7.199834e-05 9.999743e-01 0.000026
4 5 6.563219 1.041079e-02 9.951398e-01 0.004860
5 6 7.690930 5.549902e-03 9.973851e-01 0.002615
6 7 1.448735 2.287310e-01 8.858561e-01 0.114144
7 8 6.989473 8.199050e-03 9.961485e-01 0.003852
8 9 2.628779 1.049424e-01 9.478637e-01 0.052136
9 10 3.128995 7.691114e-02 3.817921e-02 0.961821
10 11 24.977930 5.799032e-07 4.353127e-08 1.000000
The expected value solution applied to scenario 2 satisfies neither the min_bin_size
constraint nor the monotonicity constraint, hence the solution is not feasible.
[50]:
EVS_2 = -np.inf
Expected value of perfect information (EVPI)¶
If we have prior information about the incoming economic scenarios, we could take optimal solutions for each scenario, with total IV:
[51]:
DIV0 = optb0.binning_table.iv
DIV1 = optb1.binning_table.iv
DIV2 = optb2.binning_table.iv
DIV = (DIV0 + DIV1 + DIV2) / 3
[52]:
DIV
[52]:
0.9990011753826167
However, this information is unlikely to be available in advance, so the best we can do in the long run is to use the stochastic programming, with expected total IV:
[53]:
SIV = sboptb.binning_table.iv
[54]:
SIV
[54]:
0.38426601503532376
The difference, in the case of perfect information, is the expected value of perfect information (EVPI) given by:
[55]:
EVPI = DIV - SIV
EVPI
[55]:
0.6147351603472929
Value of stochastic solution (VSS)¶
The loss in IV by not considering stochasticity is the difference between the application of the expected value solution for each scenario and the stochastic model IV. The application of the EVS to each scenario results in infeasible solutions, thus
[56]:
VSS = SIV - (EVS_0 + EVS_1 + EVS_2)
VSS
[56]:
inf