Tutorial: FICO Explainable Machine Learning Challenge¶
In this tutorial, we use the dataset form the FICO Explainable Machine Learning Challenge: https://community.fico.com/s/explainable-machine-learning-challenge. The goal is to create a pipeline by combining a binning process and logistic regression to obtain an explainable model and compare it against a black-box model using Gradient Boosting Tree (GBT) as an estimator.
[1]:
import matplotlib.pyplot as plt
import numpy as np
import pandas as pd
[2]:
from optbinning import BinningProcess
from sklearn.linear_model import LogisticRegression
from sklearn.ensemble import GradientBoostingClassifier
from sklearn.metrics import classification_report
from sklearn.metrics import auc, roc_auc_score, roc_curve
from sklearn.model_selection import train_test_split
from sklearn.pipeline import Pipeline
Download the dataset from the link above and load it.
[3]:
df = pd.read_csv("data/FICO_challenge/heloc_dataset_v1.csv", sep=",")
[4]:
variable_names = list(df.columns[1:])
[5]:
X = df[variable_names].values
Transform the categorical dichotomic target variable into numerical.
[6]:
y = df.RiskPerformance.values
mask = y == "Bad"
y[mask] = 1
y[~mask] = 0
y = y.astype(int)
Modeling¶
The data dictionary of this challenge includes three special values/codes:
-9 No Bureau Record or No Investigation
-8 No Usable/Valid Trades or Inquiries
-7 Condition not Met (e.g. No Inquiries, No Delinquencies)
[7]:
special_codes = [-9, -8, -7]
This challenge imposes monotonicity constraints with respect to the probability of a bad target for many of the variables. We apply these rules by passing the following dictionary of parameters for these variables involved.
[8]:
binning_fit_params = {
"ExternalRiskEstimate": {"monotonic_trend": "descending"},
"MSinceOldestTradeOpen": {"monotonic_trend": "descending"},
"MSinceMostRecentTradeOpen": {"monotonic_trend": "descending"},
"AverageMInFile": {"monotonic_trend": "descending"},
"NumSatisfactoryTrades": {"monotonic_trend": "descending"},
"NumTrades60Ever2DerogPubRec": {"monotonic_trend": "ascending"},
"NumTrades90Ever2DerogPubRec": {"monotonic_trend": "ascending"},
"PercentTradesNeverDelq": {"monotonic_trend": "descending"},
"MSinceMostRecentDelq": {"monotonic_trend": "descending"},
"NumTradesOpeninLast12M": {"monotonic_trend": "ascending"},
"MSinceMostRecentInqexcl7days": {"monotonic_trend": "descending"},
"NumInqLast6M": {"monotonic_trend": "ascending"},
"NumInqLast6Mexcl7days": {"monotonic_trend": "ascending"},
"NetFractionRevolvingBurden": {"monotonic_trend": "ascending"},
"NetFractionInstallBurden": {"monotonic_trend": "ascending"},
"NumBank2NatlTradesWHighUtilization": {"monotonic_trend": "ascending"}
}
Instantiate a BinningProcess
object class with variable names, special codes and dictionary of binning parameters. Create a explainable model pipeline and a black-blox pipeline.
[9]:
binning_process = BinningProcess(variable_names, special_codes=special_codes,
binning_fit_params=binning_fit_params)
[10]:
clf1 = Pipeline(steps=[('binning_process', binning_process),
('classifier', LogisticRegression(solver="lbfgs"))])
clf2 = LogisticRegression(solver="lbfgs")
clf3 = GradientBoostingClassifier()
Split dataset into train and test. Fit pipelines with training data, then generate classification reports to show the main classification metrics.
[11]:
X_train, X_test, y_train, y_test = train_test_split(X, y, test_size=0.2, random_state=42)
[12]:
clf1.fit(X_train, y_train)
/home/gui/projects/github/top/optbinning/optbinning/binning/transformations.py:38: RuntimeWarning: invalid value encountered in log
return np.log((1. / event_rate - 1) * n_event / n_nonevent)
[12]:
Pipeline(steps=[('binning_process',
BinningProcess(binning_fit_params={'AverageMInFile': {'monotonic_trend': 'descending'},
'ExternalRiskEstimate': {'monotonic_trend': 'descending'},
'MSinceMostRecentDelq': {'monotonic_trend': 'descending'},
'MSinceMostRecentInqexcl7days': {'monotonic_trend': 'descending'},
'MSinceMostRecentTradeOpen': {'monotonic_trend': 'descen...
'MaxDelqEver', 'NumTotalTrades',
'NumTradesOpeninLast12M',
'PercentInstallTrades',
'MSinceMostRecentInqexcl7days',
'NumInqLast6M',
'NumInqLast6Mexcl7days',
'NetFractionRevolvingBurden',
'NetFractionInstallBurden',
'NumRevolvingTradesWBalance',
'NumInstallTradesWBalance',
'NumBank2NatlTradesWHighUtilization',
'PercentTradesWBalance'])),
('classifier', LogisticRegression())])
[13]:
clf2.fit(X_train, y_train)
/home/gui/anaconda3/lib/python3.7/site-packages/sklearn/linear_model/_logistic.py:818: ConvergenceWarning: lbfgs failed to converge (status=1):
STOP: TOTAL NO. of ITERATIONS REACHED LIMIT.
Increase the number of iterations (max_iter) or scale the data as shown in:
https://scikit-learn.org/stable/modules/preprocessing.html
Please also refer to the documentation for alternative solver options:
https://scikit-learn.org/stable/modules/linear_model.html#logistic-regression
extra_warning_msg=_LOGISTIC_SOLVER_CONVERGENCE_MSG,
[13]:
LogisticRegression()
[14]:
clf3.fit(X_train, y_train)
[14]:
GradientBoostingClassifier()
[15]:
y_pred = clf1.predict(X_test)
print(classification_report(y_test, y_pred))
precision recall f1-score support
0 0.70 0.66 0.68 1004
1 0.70 0.74 0.72 1088
accuracy 0.70 2092
macro avg 0.70 0.70 0.70 2092
weighted avg 0.70 0.70 0.70 2092
/home/gui/projects/github/top/optbinning/optbinning/binning/transformations.py:38: RuntimeWarning: invalid value encountered in log
return np.log((1. / event_rate - 1) * n_event / n_nonevent)
[16]:
y_pred = clf2.predict(X_test)
print(classification_report(y_test, y_pred))
precision recall f1-score support
0 0.67 0.66 0.67 1004
1 0.69 0.70 0.70 1088
accuracy 0.68 2092
macro avg 0.68 0.68 0.68 2092
weighted avg 0.68 0.68 0.68 2092
[17]:
y_pred = clf3.predict(X_test)
print(classification_report(y_test, y_pred))
precision recall f1-score support
0 0.71 0.66 0.68 1004
1 0.70 0.75 0.73 1088
accuracy 0.71 2092
macro avg 0.71 0.70 0.70 2092
weighted avg 0.71 0.71 0.70 2092
Plot the Receiver Operating Characteristic (ROC) metric to evaluate and compare the classifiers’ prediction.
[18]:
probs = clf1.predict_proba(X_test)
preds = probs[:,1]
fpr1, tpr1, threshold = roc_curve(y_test, preds)
roc_auc1 = auc(fpr1, tpr1)
probs = clf2.predict_proba(X_test)
preds = probs[:,1]
fpr2, tpr2, threshold = roc_curve(y_test, preds)
roc_auc2 = auc(fpr2, tpr2)
probs = clf3.predict_proba(X_test)
preds = probs[:,1]
fpr3, tpr3, threshold = roc_curve(y_test, preds)
roc_auc3 = auc(fpr3, tpr3)
/home/gui/projects/github/top/optbinning/optbinning/binning/transformations.py:38: RuntimeWarning: invalid value encountered in log
return np.log((1. / event_rate - 1) * n_event / n_nonevent)
[19]:
plt.title('Receiver Operating Characteristic')
plt.plot(fpr1, tpr1, 'b', label='Binning+LR: AUC = {0:.2f}'.format(roc_auc1))
plt.plot(fpr2, tpr2, 'g', label='LR: AUC = {0:.2f}'.format(roc_auc2))
plt.plot(fpr3, tpr3, 'r', label='GBT: AUC = {0:.2f}'.format(roc_auc3))
plt.legend(loc='lower right')
plt.plot([0, 1], [0, 1],'k--')
plt.xlim([0, 1])
plt.ylim([0, 1])
plt.ylabel('True Positive Rate')
plt.xlabel('False Positive Rate')
plt.show()
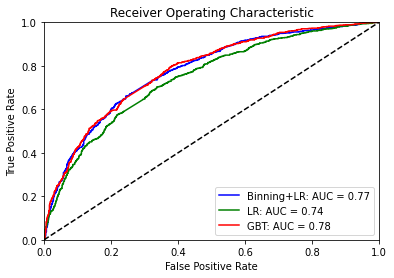
The plot above shows the increment in terms of model performance after binning when the logistic estimator is chosen. Furthermore, a previous binning process might reduce numerical instability issues, as confirmed when fitting the classifier clf2
.
Binning process statistics¶
The binning process of the pipeline can be retrieved to show information about the problem and timing statistics.
[20]:
binning_process.information(print_level=2)
optbinning (Version 0.19.0)
Copyright (c) 2019-2024 Guillermo Navas-Palencia, Apache License 2.0
Begin options
max_n_prebins 20 * d
min_prebin_size 0.05 * d
min_n_bins no * d
max_n_bins no * d
min_bin_size no * d
max_bin_size no * d
max_pvalue no * d
max_pvalue_policy consecutive * d
selection_criteria no * d
fixed_variables no * d
categorical_variables no * d
special_codes yes * U
split_digits no * d
binning_fit_params yes * U
binning_transform_params no * d
verbose False * d
End options
Statistics
Number of records 8367
Number of variables 23
Target type binary
Number of numerical 23
Number of categorical 0
Number of selected 23
Time 2.3069 sec
The summary
method returns basic statistics for each binned variable.
[21]:
binning_process.summary()
[21]:
name | dtype | status | selected | n_bins | iv | js | gini | quality_score | |
---|---|---|---|---|---|---|---|---|---|
0 | ExternalRiskEstimate | numerical | OPTIMAL | True | 12 | 1.018368 | 0.116638 | 0.534387 | 0.031792 |
1 | MSinceOldestTradeOpen | numerical | OPTIMAL | True | 11 | 0.252786 | 0.030483 | 0.264740 | 0.027179 |
2 | MSinceMostRecentTradeOpen | numerical | OPTIMAL | True | 6 | 0.019086 | 0.002377 | 0.065597 | 0.000556 |
3 | AverageMInFile | numerical | OPTIMAL | True | 10 | 0.319379 | 0.038458 | 0.304157 | 0.128082 |
4 | NumSatisfactoryTrades | numerical | OPTIMAL | True | 10 | 0.126726 | 0.015424 | 0.180888 | 0.001210 |
5 | NumTrades60Ever2DerogPubRec | numerical | OPTIMAL | True | 4 | 0.178710 | 0.021915 | 0.200184 | 0.201631 |
6 | NumTrades90Ever2DerogPubRec | numerical | OPTIMAL | True | 3 | 0.133485 | 0.016301 | 0.155193 | 0.286527 |
7 | PercentTradesNeverDelq | numerical | OPTIMAL | True | 8 | 0.377803 | 0.045428 | 0.316946 | 0.101421 |
8 | MSinceMostRecentDelq | numerical | OPTIMAL | True | 7 | 0.289526 | 0.035246 | 0.272229 | 0.239494 |
9 | MaxDelq2PublicRecLast12M | numerical | OPTIMAL | True | 3 | 0.330280 | 0.040250 | 0.301670 | 0.833712 |
10 | MaxDelqEver | numerical | OPTIMAL | True | 4 | 0.236098 | 0.029129 | 0.257314 | 0.667940 |
11 | NumTotalTrades | numerical | OPTIMAL | True | 8 | 0.064716 | 0.008027 | 0.138545 | 0.011755 |
12 | NumTradesOpeninLast12M | numerical | OPTIMAL | True | 6 | 0.023530 | 0.002936 | 0.083770 | 0.007932 |
13 | PercentInstallTrades | numerical | OPTIMAL | True | 8 | 0.098610 | 0.012107 | 0.159569 | 0.077405 |
14 | MSinceMostRecentInqexcl7days | numerical | OPTIMAL | True | 4 | 0.166538 | 0.020460 | 0.211639 | 0.531041 |
15 | NumInqLast6M | numerical | OPTIMAL | True | 4 | 0.089956 | 0.011127 | 0.159369 | 0.323780 |
16 | NumInqLast6Mexcl7days | numerical | OPTIMAL | True | 5 | 0.083992 | 0.010394 | 0.153641 | 0.036291 |
17 | NetFractionRevolvingBurden | numerical | OPTIMAL | True | 9 | 0.574686 | 0.068232 | 0.410605 | 0.343593 |
18 | NetFractionInstallBurden | numerical | OPTIMAL | True | 5 | 0.037879 | 0.004724 | 0.105916 | 0.053723 |
19 | NumRevolvingTradesWBalance | numerical | OPTIMAL | True | 7 | 0.093376 | 0.011578 | 0.162108 | 0.011291 |
20 | NumInstallTradesWBalance | numerical | OPTIMAL | True | 5 | 0.014121 | 0.001762 | 0.059437 | 0.010423 |
21 | NumBank2NatlTradesWHighUtilization | numerical | OPTIMAL | True | 5 | 0.334853 | 0.041017 | 0.308402 | 0.222126 |
22 | PercentTradesWBalance | numerical | OPTIMAL | True | 12 | 0.365412 | 0.044210 | 0.334112 | 0.018131 |
The get_binned_variable
method serves to retrieve an optimal binning object, which can be analyzed in detail afterward.
[22]:
optb = binning_process.get_binned_variable("NumBank2NatlTradesWHighUtilization")
[23]:
optb.binning_table.build()
[23]:
Bin | Count | Count (%) | Non-event | Event | Event rate | WoE | IV | JS | |
---|---|---|---|---|---|---|---|---|---|
0 | (-inf, 0.50) | 3416 | 0.408271 | 2184 | 1232 | 0.360656 | 0.662217 | 0.175281 | 0.021518 |
1 | [0.50, 1.50) | 2015 | 0.240827 | 858 | 1157 | 0.574194 | -0.209284 | 0.010461 | 0.001305 |
2 | [1.50, 2.50) | 983 | 0.117485 | 345 | 638 | 0.649034 | -0.525096 | 0.031309 | 0.003869 |
3 | [2.50, 3.50) | 496 | 0.059281 | 137 | 359 | 0.723790 | -0.873644 | 0.041802 | 0.005065 |
4 | [3.50, inf) | 521 | 0.062268 | 139 | 382 | 0.733205 | -0.921249 | 0.048466 | 0.005853 |
5 | Special | 936 | 0.111868 | 333 | 603 | 0.644231 | -0.504077 | 0.027533 | 0.003406 |
6 | Missing | 0 | 0.000000 | 0 | 0 | 0.000000 | 0.000000 | 0.000000 | 0.000000 |
Totals | 8367 | 1.000000 | 3996 | 4371 | 0.522409 | 0.334853 | 0.041017 |
[24]:
optb.binning_table.plot(metric="event_rate")
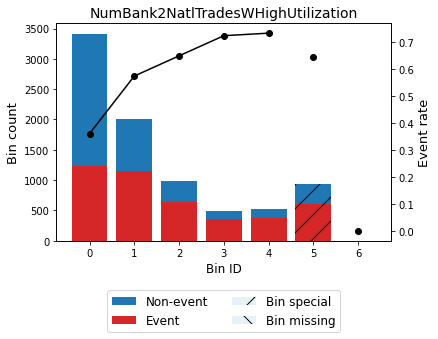
[25]:
optb.binning_table.analysis()
---------------------------------------------
OptimalBinning: Binary Binning Table Analysis
---------------------------------------------
General metrics
Gini index 0.30840174
IV (Jeffrey) 0.33485338
JS (Jensen-Shannon) 0.04101657
Hellinger 0.04143062
Triangular 0.16088993
KS 0.26468885
HHI 0.25839135
HHI (normalized) 0.13478991
Cramer's V 0.28612357
Quality score 0.22212566
Monotonic trend ascending
Significance tests
Bin A Bin B t-statistic p-value P[A > B] P[B > A]
0 1 234.556206 6.049946e-53 5.576352e-107 1.000000
1 2 15.402738 8.686234e-05 7.665294e-06 0.999992
2 3 8.386138 3.780934e-03 1.290940e-03 0.998709
3 4 0.113909 7.357368e-01 3.678894e-01 0.632111